一、场景
-
业务场景
-
- 性能、成本的强烈优化诉求:历史数据的长周期保存极大影响了业务查询性能,同时因为空间的持续膨胀,PG集群规格持续垂直扩容,造成了计算资源的浪费
- 历史库方案成本高,效果差:尝试通过独立在线库和历史库方案进行历史数据归档的工作,但是因为对于历史数据存在更新和查询场景,始终无法在核心业务投产
- 根据业务场景的一些特性,需要对商家及特定活动时进行资源调整
-
测试场景
二、测试预期
- 预期性能描述:
-
- 写入10W rps/s
-
- 查询平均rt在100毫秒级别
三、硬件配置
- 1台FE: 4vCPU 16GiB,通用型 g6
- 5台BE:16 vCPU 128GiB,高主频内存型 hfr7
- 网络带宽:10Gbps
- 存储:2.5T
- 版本:2.5.2&&3.1
四、其他条件
- 调整参数:
lake_gc_metadata_max_versions = 3
lake_gc_metadata_check_interval = 60
lake_gc_segment_check_interval = 60
experimental_lake_enable_fast_gc = true
lake_gc_segment_expire_seconds = 3600
enable_storage_cache = true&&false
disable_storage_page_cache=true
storage_cache_ttl=2592000
五、测试结果
1. 写入(stream load)
- Flink任务4并发度、4.25CPU、17G内存的情况下不同场景的写入表现情况,
- 基础表结构如下:
CREATE TABLE `dwd_jst_erp_trd_order_order_item_split_combinesku_ri_pk_cache_async` ( `co_id` int(11) NOT NULL COMMENT "", `o_id` bigint(20) NOT NULL COMMENT "", `oi_id` bigint(20) NOT NULL COMMENT "", `dw_oi_sku_id_md5` varchar(32) NOT NULL COMMENT "", `co_key` int(11) NOT NULL COMMENT "", `dw_oi_sku_id` varchar(65533) NOT NULL COMMENT "", `warehouse_id` int(11) NOT NULL COMMENT "", `warehouse_name` varchar(65533) NULL COMMENT "", `status` varchar(65533) NULL COMMENT "", `dw_oi_qty` bigint(20) NOT NULL COMMENT "", `dw_oi_item_pay_amount_split` decimal128(38, 4) NOT NULL COMMENT "", `plan_delivery_date_fmt` datetime NOT NULL COMMENT "", `labels` varchar(65533) NOT NULL COMMENT "", `shop_id` int(11) NULL COMMENT "", `shop_name` varchar(65533) NULL COMMENT "", `platform_cn` varchar(65533) NULL COMMENT "", `distr_id` int(11) NULL COMMENT "", `distr_name` varchar(65533) NULL COMMENT "", `shared_version` int(11) NOT NULL COMMENT "", `pay_date` datetime NOT NULL COMMENT "", `send_date` datetime NULL COMMENT "", `invalarm_active` smallint(6) NOT NULL COMMENT "", `flink_modified` datetime NOT NULL COMMENT "", INDEX bitmap_index_invalarm_active (`invalarm_active`) USING BITMAP COMMENT 'invalarm_active作为bitmap索引列', INDEX bitmap_index_status (`status`) USING BITMAP COMMENT 'status作为bitmap索引列') ENGINE=OLAP PRIMARY KEY(`co_id`, `o_id`, `oi_id`, `dw_oi_sku_id_md5`, `co_key`)COMMENT "开启本地缓存,开启异步"PARTITION BY RANGE(`co_key`)(PARTITION p0 VALUES [("0"), ("1")),PARTITION p1 VALUES [("1"), ("2")),PARTITION p2 VALUES [("2"), ("3")),PARTITION p3 VALUES [("3"), ("4")),PARTITION p4 VALUES [("4"), ("5")),PARTITION p5 VALUES [("5"), ("6")),PARTITION p6 VALUES [("6"), ("7")),PARTITION p7 VALUES [("7"), ("8")),PARTITION p8 VALUES [("8"), ("9")),PARTITION p9 VALUES [("9"), ("10")),PARTITION p10 VALUES [("10"), ("11")),PARTITION p11 VALUES [("11"), ("12")),PARTITION p12 VALUES [("12"), ("13")),PARTITION p13 VALUES [("13"), ("14")),PARTITION p14 VALUES [("14"), ("15")),PARTITION p15 VALUES [("15"), ("16")),PARTITION p16 VALUES [("16"), ("17")),PARTITION p17 VALUES [("17"), ("18")),PARTITION p18 VALUES [("18"), ("19")),PARTITION p19 VALUES [("19"), ("20")),PARTITION p20 VALUES [("20"), ("21")),PARTITION p21 VALUES [("21"), ("22")),PARTITION p22 VALUES [("22"), ("23")),PARTITION p23 VALUES [("23"), ("24")),PARTITION p24 VALUES [("24"), ("25")),PARTITION p25 VALUES [("25"), ("26")),PARTITION p26 VALUES [("26"), ("27")),PARTITION p27 VALUES [("27"), ("28")),PARTITION p28 VALUES [("28"), ("29")),PARTITION p29 VALUES [("29"), ("30")),PARTITION p30 VALUES [("30"), ("31")),PARTITION p31 VALUES [("31"), ("32")))DISTRIBUTED BY HASH(`co_id`, `o_id`, `oi_id`) BUCKETS 2 PROPERTIES ("replication_num" = "1","bloom_filter_columns" = "dw_oi_sku_id","enable_storage_cache" = "true","storage_cache_ttl" = "2592000","enable_async_write_back" = "false","compression" = "LZ4");
a. 存算分离主键表开启本地缓存,开启异步写入对象存储
参数:
enable_storage_cache = true,
storage_cache_ttl = 2592000,
enable_async_write_back = true
平均20K左右
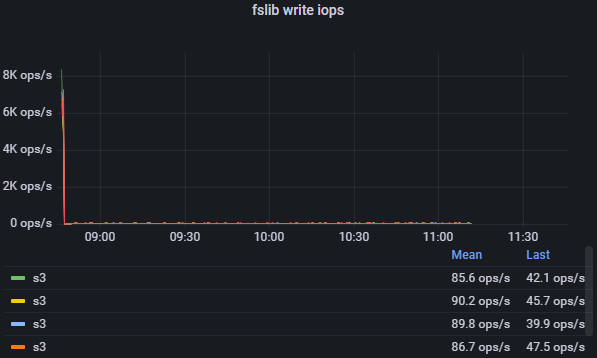
写IO平均90 ops/s
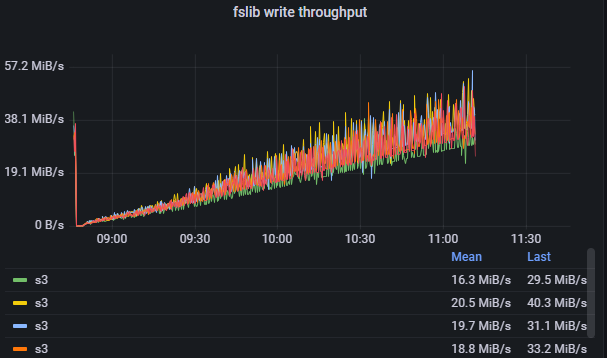
写吞吐17MiB/s
b. 存算分离主键表开启本地缓存,关闭异步写入对象存储
参数:
enable_storage_cache = true,
storage_cache_ttl = 2592000,
enable_async_write_back = false
平均3K
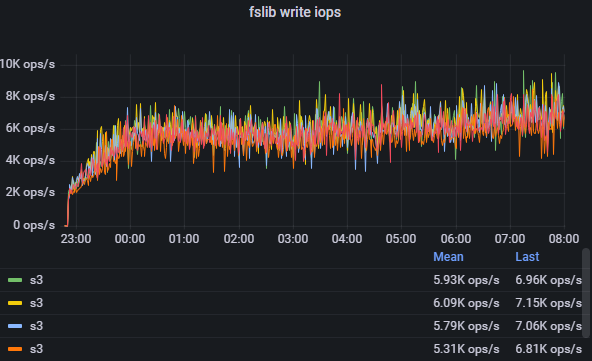
写IO平均5.7K ops/s
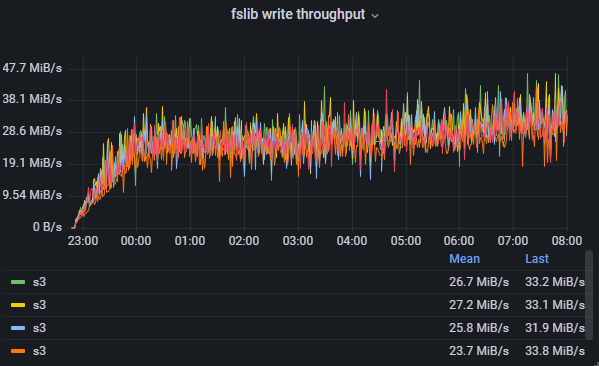
写吞吐平均24MiB/s
c. 存算分离主键表关闭本地缓存
参数:
enable_storage_cache = false,
storage_cache_ttl = 0,
enable_async_write_back = false
平均28K左右
d. 存算分离明细表关闭本地缓存
参数:
enable_storage_cache = false,
storage_cache_ttl = 0,
enable_async_write_back = false
平均20K左右
e. 存算一体主键表
平均25K
2. 查询
分别测试在业务场景下单SQL表现及根据上线需求回放5000个SQL的表现
表数据量3.67亿,存储18G左右
查询SQL如下:
select shop_id, distributor_id, shop_name, distributor_name, platform, no_sent_qty , time_out_sent_qty, will_sent_qty_1d, will_sent_qty_1d_3d, will_sent_qty_3d_7d, will_sent_qty_7dfrom ( select shop_id, null as distributor_id, max(shop_name) as shop_name, null as distributor_name, max(platform_cn) as platform, sum(dw_oi_qty) as no_sent_qty , sum(case when plan_delivery_date_fmt<now() then dw_oi_qty else 0 end) as time_out_sent_qty, sum(case when plan_delivery_date_fmt>=now() and plan_delivery_date_fmt< DATE_ADD(now(), INTERVAL 1 DAY) then dw_oi_qty else 0 end) as will_sent_qty_1d, sum(case when plan_delivery_date_fmt>= DATE_ADD(now(), INTERVAL 1 DAY) and plan_delivery_date_fmt< DATE_ADD(now(), INTERVAL 3 DAY) then dw_oi_qty else 0 end) as will_sent_qty_1d_3d, sum(case when plan_delivery_date_fmt>= DATE_ADD(now(), INTERVAL 3 DAY) and plan_delivery_date_fmt< DATE_ADD(now(), INTERVAL 7 DAY) then dw_oi_qty else 0 end) as will_sent_qty_3d_7d, sum(case when plan_delivery_date_fmt>= DATE_ADD(now(), INTERVAL 7 DAY) then dw_oi_qty else 0 end) as will_sent_qty_7d from dwd_jst_erp_trd_order_order_item_split_combinesku_ri_pk_cache_async where co_id=10002948 and co_key = 4 and invalarm_active = 1 and pay_date>=DATE_ADD(CURRENT_DATE, INTERVAL -29 DAY) and status in ("WAITCONFIRM","WAITFCONFIRM","WAITDELIVER","DELIVERING","QUESTION","WAITOUTERSENT") and distr_id is null group by shop_id union all select null as shop_id, distr_id as distributor_id, null as shop_name, max(distr_name) as distributor_name, null as platform, sum(dw_oi_qty) as no_sent_qty , sum(case when plan_delivery_date_fmt<now() then dw_oi_qty else 0 end) as time_out_sent_qty, sum(case when plan_delivery_date_fmt>=now() and plan_delivery_date_fmt< DATE_ADD(now(), INTERVAL 1 DAY) then dw_oi_qty else 0 end) as will_sent_qty_1d, sum(case when plan_delivery_date_fmt>= DATE_ADD(now(), INTERVAL 1 DAY) and plan_delivery_date_fmt< DATE_ADD(now(), INTERVAL 3 DAY) then dw_oi_qty else 0 end) as will_sent_qty_1d_3d, sum(case when plan_delivery_date_fmt>= DATE_ADD(now(), INTERVAL 3 DAY) and plan_delivery_date_fmt< DATE_ADD(now(), INTERVAL 7 DAY) then dw_oi_qty else 0 end) as will_sent_qty_3d_7d, sum(case when plan_delivery_date_fmt>= DATE_ADD(now(), INTERVAL 7 DAY) then dw_oi_qty else 0 end) as will_sent_qty_7d from dwd_jst_erp_trd_order_order_item_split_combinesku_ri_pk_cache_async where co_id=10002948 and co_key = 4 and invalarm_active = 1 and pay_date>=DATE_ADD(CURRENT_DATE, INTERVAL -29 DAY) and status in ("WAITCONFIRM","WAITFCONFIRM","WAITDELIVER","DELIVERING","QUESTION","WAITOUTERSENT") and distr_id is not null group by distr_id) torder by "no_sent_qty" asc, shop_id,distributor_id;
a. 存算一体本地表
参数:
disable_storage_page_cache=false
查询profile:
测试方式:测试50秒发送5000个SQL的耗时表现,耗时=48.22, QPS≈103.69
2023-05-26 16:43:12,528 - sr_sql_stress.py[line:155] - INFO: 执行结束:计划时间为2023-03-22 16:30:10,任务实际开始时间为2023-05-26 16:43:11,总计花费0.780968毫秒,对应下标4931,程序开始时间为2023-05-26 16:42:22.889387
b. 存算一体外部表开启本地缓存
参数:
enable_populate_block_cache = true
block_cache_enable=true
目前文件外部表只支持设置parquet和orc场景,对于当前现有集群搬迁数据较不适用,暂不测试此种场景
c. 存算分离开启本地缓存主键表
参数:
enable_storage_cache = true
storage_cache_ttl = 2592000
查询profile:
ReadLocalDisk:925.25KB , ReadRemote:0 从本地磁盘读的大小为925.25KB,从远程存储读的为0,全部走本地缓存查询,符合预期
测试方式:测试50秒发送5000个SQL的耗时表现,耗时=45.67, QPS≈109.48
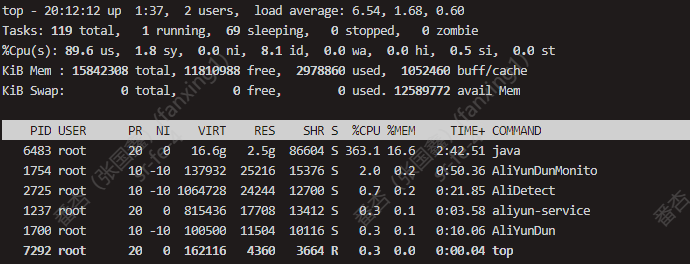
2023-05-27 00:09:08,728 - sr_sql_stress.py[line:155] - INFO: 执行结束:计划时间为2023-03-22 16:30:07,任务实际开始时间为2023-05-27 00:08:02,总计花费0.17757毫秒,对应下标4606,程序开始时间为2023-05-27 00:07:16.338853
d. 存算分离关闭本地缓存主键表
参数:
enable_storage_cache = false
storage_cache_ttl = 0
disable_storage_page_cache=true
查询profile:
ReadLocalDisk:0 , ReadRemote:3.03MB 从本地磁盘读的大小为0,从远程存储读的为3.03MB,全部走外部存储查询,符合预期
测试方式:测试50秒发送5000个SQL的耗时表现,耗时=46.49, QPS≈107.55
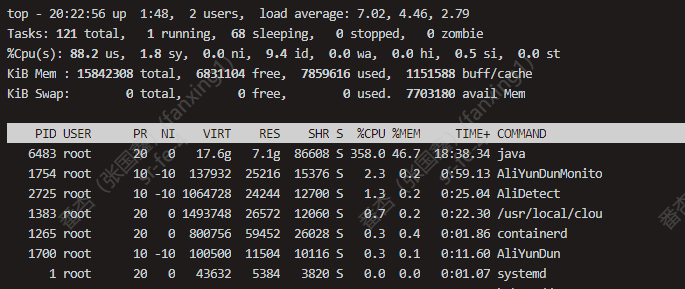
2023-05-25 20:23:36,437 - sr_sql_stress.py[line:155] - INFO: 执行结束:计划时间为2023-03-22 16:30:08,任务实际开始时间为2023-05-25 20:22:35,总计花费0.480133毫秒,对应下标4720,程序开始时间为2023-05-25 20:21:48.517708
e. 存算分离关闭本地缓存明细表
参数:
enable_storage_cache = false
storage_cache_ttl = 0
disable_storage_page_cache=true
查询profile:
测试方式:测试50秒发送5000个SQL的耗时表现,耗时=47.59, QPS≈105.06
2023-05-25 20:30:21,357 - sr_sql_stress.py[line:155] - INFO: 执行结束:计划时间为2023-03-22 16:30:09,任务实际开始时间为2023-05-25 20:29:48,总计花费1.029017毫秒,对应下标4820,程序开始时间为2023-05-25 20:29:00.419744
六、结论
1.写入场景
异步写入参数对于写入性能有较大影响,关闭后对于写入性能有大约7倍左右的提升
关闭本地缓存后,直接写入数据到oss,写入瓶颈点由oss控制
资源稳定的情况下,存算分离开启本地缓存和存算一体写入基本持平
2.内存消耗情况
存算分离的表内存消耗约为20%,对于存算一体的表来说内存消耗为50%,通过现象来看存放分离对于内存的管理更加高效
3.单SQL查询情况
对于单SQL查询来看,开启本地缓存后有明显优化,查询性能基本和存算一体表持平,根据回放测试表现来看,基本都可以符合在100左右的QPS,对于当前的业务场景基本符合预期
七、问题
1. 数据膨胀
数据写入41839881条,存储1.7G,OSS存储700多G,本地存储150*5多G
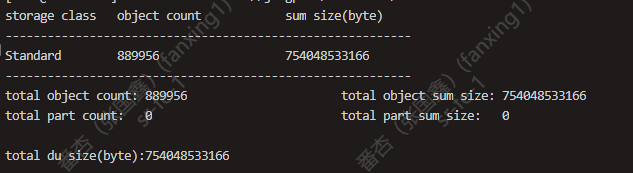
flink在写入过程中,5秒一个批次,因而compaction的次数过多,数据膨胀过多,在当前的版本中数据的过期时间是24小时,因此无法同时参数的设置来进行数据的清理,已和官方确认后续会在内核中进行优化,用户可以无感知
2. 表不可查
flink任务写入过程中调整写入并发后导致compaction的事务过多,表暂时不可查
和官方确认后,需要手动调整compaction相对应的参数来避免大批量导入过程中表不可查的情况
八、规划
目前,已根据现有的业务进行了验证,对于存算分离的版本基本可以满足当前业务场景,后续可以进行投产使用。同时会结合后续的规划及进展对存算分离的版本进行不断的测试和线上业务的实践,期待社区和官方可以更好的场景尝试及功能发布。